Renewable energies remain the main response to curb global warming. However, there is still a long way to go to achieve total renewable energy consumption. Several studies are underway to optimize the production of clean energy that can cover the world's energy consumption. To achieve this, the artificial neural network (ANN) approach, which is an artificial intelligence (AI) model, is an asset to consider. This paper aims to improve the activation function an existing Recurrent Neural Network (RNN) to minimize the losses in solar energy generated from the solar panel during its integration into the power grid. By reducing the loss of solar energy, its production increases. The RNN is designed in VDHL language and embedded on the Field Programmable Gate Array (FPGA) board for implementation. With a quantitative approach, data collected from the solar panel are converted in 32 bits floating-point. The Neural Network (NN) is trained to reduce the loss of the input data by choosing a model an activation function of the tangent hyperbolic that delivers accuracy and less latency. The reinforcement attribute of the RNN track errors in the forward feeding of neurons (nodes) and create adjustments on the output. In summary, the energy lost with the designed artificial neural network controller is significantly lower compared to the standard solar controller. This study demonstrated that the AI approach can significantly reduce the loss of solar energy during its integration into the power grid. The research pointed out that the artificial neural network is reliable approach to optimize result of digital circuit that use FPGA.
Faculty Mentor: Dr. Xingang Fu
Department of Electrical Engineering and Computer Science
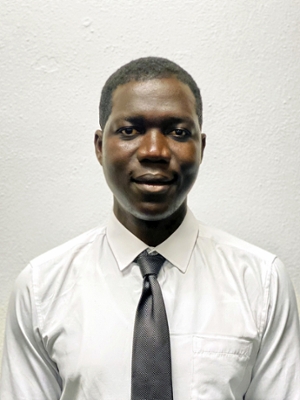